Featured Student Research
Featured Student Research
Here is a selection from our final Capstone presentations.
Using Machine Learning to Develop Real-Time Labor Predictions
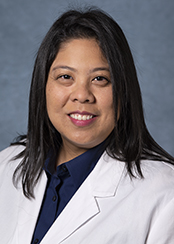
Melissa Wong, MD
Principal Investigator
For her Master of Science in Health Systems research project, Melissa Wong, MD, an assistant professor of obstetrics and gynecology and a fellow in Maternal-Fetal Medicine at Cedars-Sinai, is focused on how machine learning can predict delivery outcomes in real time.
Project Summary
There is a strong nationwide push to reduce the overall cesarean delivery rate, particularly among nulliparous, term, singleton, vertex (NTSV) patients. Part of the difficulty for providers in choosing whether or not to recommend a cesarean delivery is limited predictive utility of the available information. It would be ideal to be able to predict the likelihood of a patient achieving a vaginal delivery. The aim of this project is to develop a Partometer that would utilize intrapartum variables to predict, in real time, the risk of a patient needing a cesarean delivery.
To date, we have been successful in incorporating admission, intrapartum cervical exam, and induction agents into our model, and have generated better prediction models than currently exist in the literature. We anticipate being able to develop and test this further, and, ultimately to operationalize this to aid providers and patients in shared decision making to attempt to achieve a vaginal delivery.
If this project is successful, we should be able to aid in continuing to reduce the cesarean delivery rate while maintaining excellent maternal and neonatal outcomes. If successful, then this could be operationalized for other institutions, and become a guide for patients to safely deliver vaginally.
Key Collaborators
- Kimberly Gregory, MD, MPH – Vice Chair Women's Healthcare Quality and Performance Improvement
- Tod Davis – Associate Director, Data Sciences
- Matthew Wells – Data Scientist
Associated Laboratories and Departments
Enterprise Information Services
Data Intelligence
Have Questions or Need Help?
If you have questions or wish to learn more about the MSHS program, please contact:
Graduate School of Biomedical Sciences
8687 Melrose Ave.
Suite G-532
West Hollywood, CA 90069