Research Areas
Developing Genetic Risk-Prediction Models Using Biobank-Linked Electronic Health Records (EHR)
Accurate prediction of disease risk based on the genetic makeup of an individual is essential for effective prevention and personalized treatment. The rich clinical phenotypes stored in EHR, together with matched patients’ genetic data, enable us to develop genetic risk-prediction models for numerous clinical phenotypes and traits. Our lab is focused on developing methods to construct and evaluate risk-prediction models that can accurately predict disease risks. The long-term goal is to develop models that are accurate, equitable to different population groups, interpretable to clinicians, and translatable into the clinical setting to improve patients’ disease diagnosis.
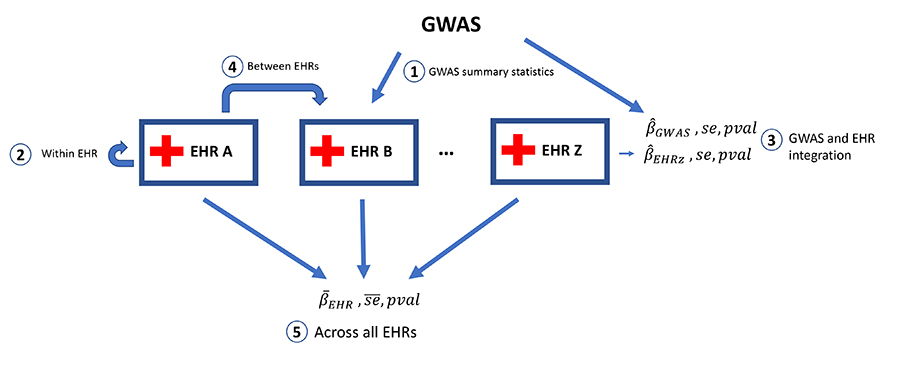
Federated Learning of EHR Data from Multiple Health Systems
An increasing number of hospitals and health systems have started to mine patients’ EHR data for novel disease-related associations. However, many of the existing analysis using EHR data has been limited to one hospital/health system at a time. An area of focus for our group is to develop methods that can extract knowledge from multiple EHR data sources, or federated learning, in order to increase the power to detect signals and improve the generalizability of results. An important consideration when integrating EHR data is that patients’ data privacy needs to be strongly protected. Thus, we will focus our efforts to develop federated learning methods that are privacy preserved.
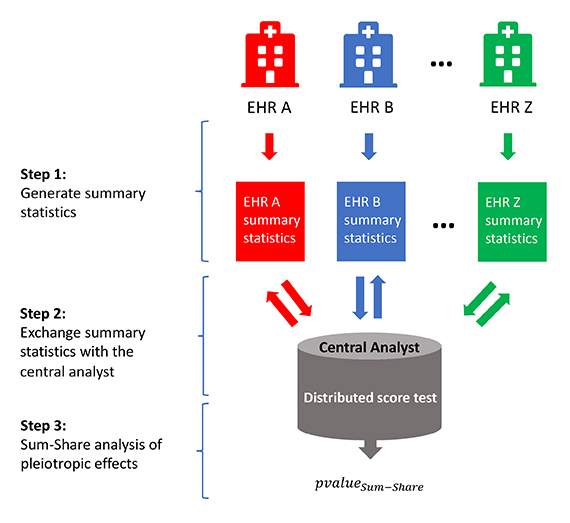
Integration of Multi-Omics Data
Perturbations across various levels of regulation, including the genome, proteome, epigenome and transcriptome, can be the causes or the effects of disease onset. These perturbations can be further modified by various environmental exposures, leading to differential disease prognosis. Thus, data integration methods are essential in identifying key risk factors and important interactions among different layers of biological systems and environmental factors to explain or predict disease risk. We are interested in developing tools to understand the complexity of diseases using multiple types of high-throughput-omics data.
Contact the R. Li Lab
Pacific Design Center
700 N. San Vicente Blvd., Suite G540
West Hollywood, CA 90069